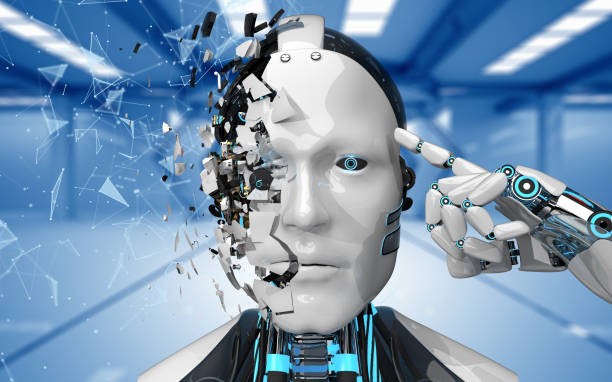
Generative AI refers to a class of machine learning algorithms that are capable of generating new content based on patterns and information that they have been trained on. The basic idea behind generative AI is to use a set of rules or parameters to create new content that resembles the original training data.
One of the most common types of generative AI is a language model. These models are typically trained on large datasets of text, such as books, articles, or social media posts. Once the model has been trained, it can be used to generate new text that is similar in style and content to the original data.
The generation process typically involves a series of steps, including text preprocessing, tokenization, embedding, and decoding. During preprocessing, the AI algorithm prepares the input data by cleaning it, removing unnecessary elements, and formatting it. Tokenization is the process of breaking down the text into individual words or phrases, while embedding involves transforming these words into numerical vectors that can be processed by the neural network. Finally, decoding involves using the AI model to generate new content based on the input data and the patterns it has learned.
There are many different approaches to generative AI, but one common method is to use a neural network. A neural network is a type of machine-learning algorithm that is modeled after the structure of the human brain. It consists of layers of interconnected nodes, each of which performs a simple calculation. By stacking multiple layers together, a neural network can perform complex tasks such as image recognition or natural language processing.
To train a generative AI model using a neural network, the first step is to input a large dataset of examples into the network. The network will then analyze the data and learn patterns and relationships between different features of the data.
Once the network has been trained, it can be used to generate new data by feeding it a random input vector. The network will then use its learned patterns and relationships to produce an output that resembles the original data. This output can be further refined and improved by adjusting the parameters of the network or by feeding it additional training data.
Generative AI has many practical applications, including natural language generation, image synthesis, and music composition. By allowing machines to generate new content based on patterns and information that they have learned from large datasets, generative AI can help to automate many creative tasks and generate new insights into complex problems.
Overall, generative AI represents an exciting and rapidly evolving field that has the potential to transform many areas of human activity. As technology continues to advance, it is likely that we will see even more sophisticated generative AI models that are capable of creating new and innovative content in a wide range of domains.
About Sysamic:
Sysamic is a modern business consulting company focused on technology, design, and business transformation. In Japan, we have over 20 years of experience supporting businesses to enter the market and accelerate growth. Our core services include eCommerce consulting and development, Business solution consulting, Microsoft Dynamics 365 Business Central, and Navision (NAV) ERP Analysis and Implementation, Infrastructure support.
At Sysamic, we value our biggest assets – our employees!
Join our Dynamics 365 team now!